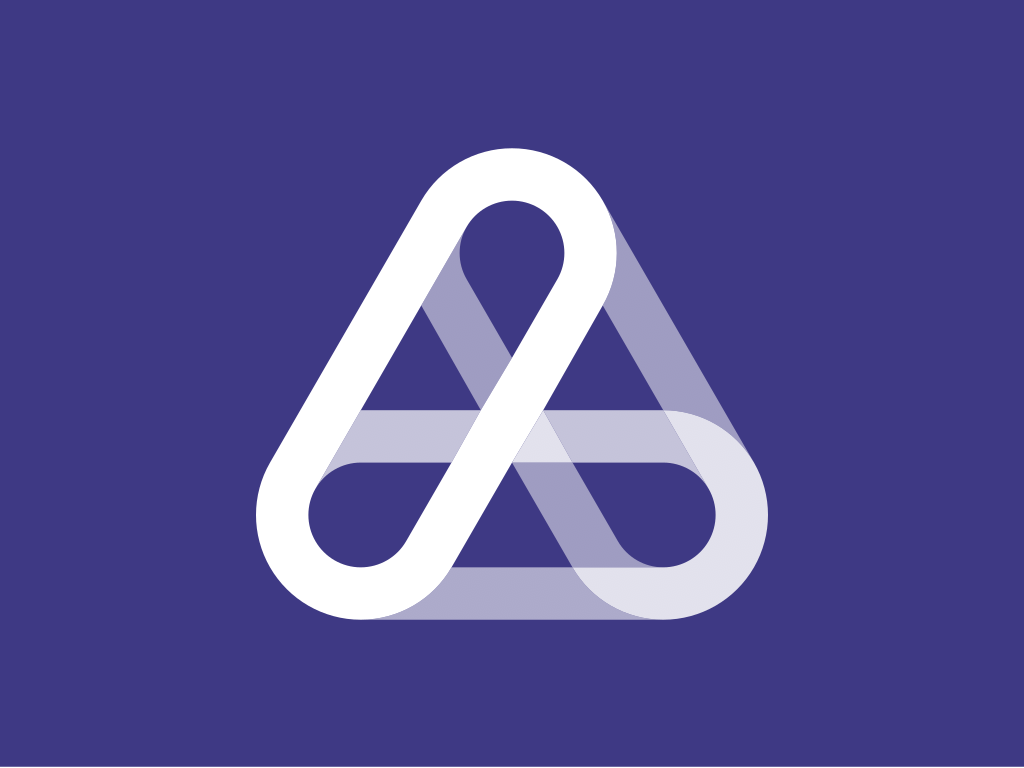
Secure data collaboration thanks to cryptographic technology
December 2022Linksight makes it easy for parties to set up secure data collaborations. To do this, we have three main pillars.
- The Linksight network, a decentralized network of data stations of connected parties such as hospitals, insurers and municipalities. These parties can easily find each other and set up as many data collaborations among themselves as they want.
- Privacy enhancing technologies (PETs) to do privacy-by-design data analysis within such a collaboration without sharing sensitive data. We use the latest PETs for this purpose, such as homomorphic encryption and secure multiparty computation (MPC).
- Data collaboration governance: setting up, the ability to enforce, and control the agreements within a data collaboration. In this way, all parties maintain control over who can do what with their data.
Setting up a data collaboration
Network participants can easily set up data collaboration with other parties. Linksight provides a so-called datastation, software that is installed in each party’s own local system or cloud. They can then connect to other organizations that also have such a datastation. We do the authentication, so each party can be sure that identity of the other is correct. From their data station, organizations can set up new data collaborations with other data stations, define the rules of those, and perform data analysis among themselves.
Encryption methods
From the toolbox of privacy technologies, we use homomorphic encryption in particular. This is an encryption method for performing analysis on data while that data remains encrypted and thus unreadable at all times. At the end of an analysis, only the end result is revealed.
Statistics
We use two types of homomorphic encryption: partial, which allows you to either add or multiply, and full (Fully HE) which can do both calculations. With partial encryption you can perform all kinds of descriptive statistics. For example, we use it in healthcare to calculate the effectiveness of interventions. With fully homomorphic encryption, much more complex calculations can be done. We use this for regression analyses, for example. For each type of analysis, we always choose the protocol that best suits it, taking into account factors such as security, speed and explainability.
Proportional disclosure
In certain cases, it is necessary to do data analysis to get a picture of certain individuals or companies. Think of fraud prevention, where you need to combine databases of agencies or banks, or to determine who is entitled to a certain benefit or compensation. For that, we use the technology private set intersection (PSI). This reveals only those individuals who meet criteria across multiple parties, without revealing all other individuals who do not meet them. Again, our “data collaboration governance “ is important, to define exactly who is allowed to do what with this data, and make that transparent and auditable.
Federated learning
For some applications, we do not use cryptographic methods, but more machine learning-based technologies to do collaborative data analysis. Here we are talking about federated learning (FL), a method to optimize data models across different parties, allowing for ever better predictions aimed at improving care or in other domains.
Data collaboration governance
Data collaboration requires clear agreements between parties and meticulous adherence to them. Our unique data collaboration governance ensures that parties maintain control over the collaboration and can technically enforce what happens within it. For example, the platform allows you to preset which party may perform which analysis under which conditions, and there are rules for statistical disclosure control. Any action outside these agreements is blocked by the system.
FAIR data
Transparency is essential here. In advance, parties provide data descriptions (FAIR) that define how their data is constructed and what their data means. This allows the other parties to understand how they can use that data, and what the quality is.
Audit trail
Transparency also applies retrospectively. Every analysis performed and every change in the rules of cooperation are immutably recorded in the decentralized network. This way it can always be proven afterwards which party performed which analysis when, under which conditions. For this decentralized audit trail, we use blockchain technology, so that parties are not dependent on Linksight for this audit trail, but it is maintained redundantly and irrefutably by different servers.